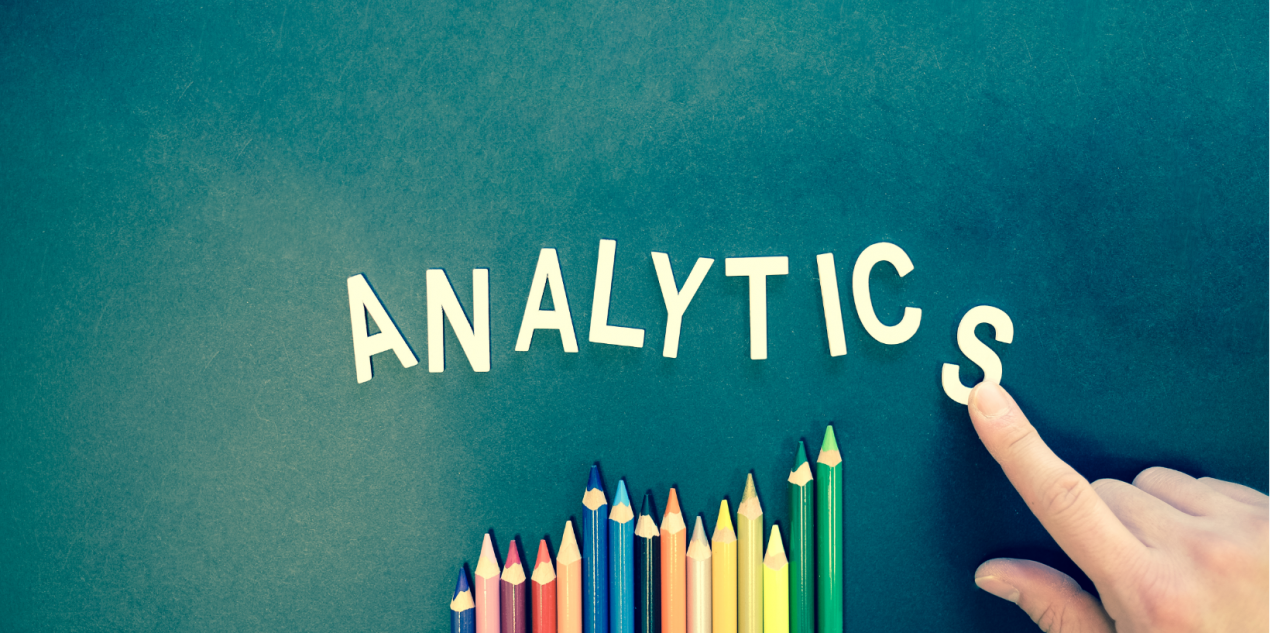
Archive article - published on March 02 2021
The currency of modern business is data. By following the data, an organization is often led down the road to riches. Data, and the intrinsic value it holds, is certainly nothing new. Dataversity.net reminds us that ancient Egyptians used statistics for building pyramids. The Roman census provided useful insight for the management of an empire. While considered an enormous undertaking for its time, historians estimate that around 80,000 citizens were counted. The magnitude of data being managed and used then is unfathomably different compared to today. The magnitude of data has grown exponentially.
According to IDC, the datasphere will grow to 175 zettabytes by 2025. Legacy data management solutions facing this surge in data won't make the cut. The rapid advances in the technologies surrounding data mean there is more need for access by more people (democratization). But, working and moving data at scale is hard and poses new challenges surrounding security, quality, and interpretability.
Rethinking the Foundation of Analytics
Data warehouses have been the custodians of enterprises' most important business data for the last 15-20 years. As enterprises are increasingly becoming data-driven, data warehouses play an extremely critical role in the digital transformation journey. As per Gartner, data warehouses often form the foundation of an enterprise's analytics strategy.
Analytics leaders and CIOs face serious challenges as the rising business demands of users and the increasing number of data streams exceed IT capacity and funding. Growing data volumes are creating IT sprawl that is complex to manage and secure. Increasing performance challenges combined with data access limitations are resulting in poor and fragmented insights. Problems stem from these typical challenges:
- Data warehouse is unable to cope-up with the ever-growing business needs
- Increasing digital initiatives creating massive data volume and flooding system
- Multiple data silos
- Data warehouse is not reflecting what's happening in your business now
- Data access limited/restricted due to performance, security, and governance challenges
- Renewing licenses and paying for expensive support resources become challenging
For many IT leaders, these challenges present a need to transform their businesses digitally. Data analytics spending and a modern data warehouse are at the heart of this transformation.
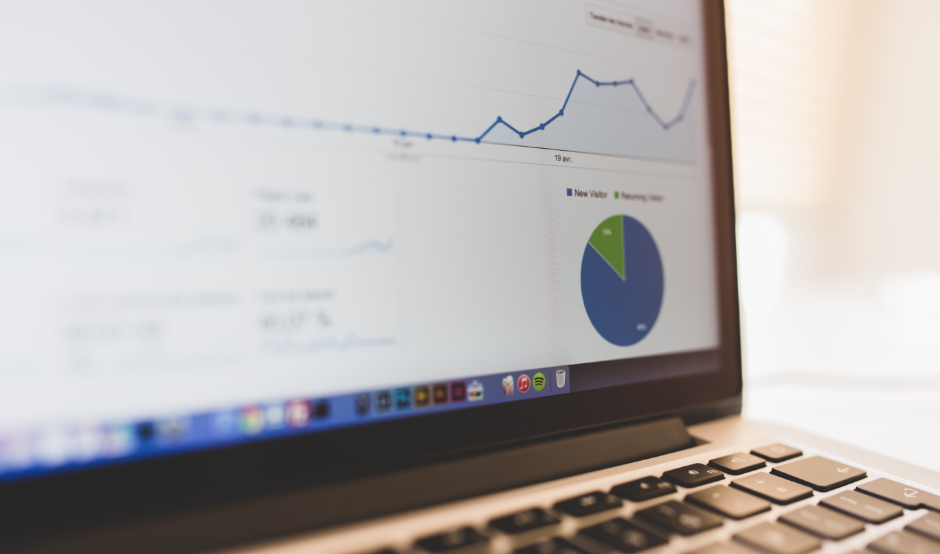
Modernizing Analytics Starts with a Pipeline
Joining disparate data sources into a single data lake is a formidable challenge. Google Cloud provides the services to address ETL (extract, transform and load) and maximize value from the data stores, even as new data sources are added.
Consider this fictional scenario. Easy-Breezes Hammocks hosts its customer service platform on Amazon Web Services (AWS). The application generates scads of data which is stored in an AWS Simple Storage Service (S3). This is just one of the half-dozen data sources the company has identified. The company needs the data from all of its sources in its data lake to be analyzed by SQL-based analytics tools and be available as raw files for backup and retention purposes. Adding a level of complexity, much of the data contains personally identifiable information. Daily, all the data needs to be cleaned and joined for analysis.
In the past, a scenario like the one above would overwhelm a conventional data infrastructure. Today, with a solution like Google Cloud Data Fusion, a fully managed, cloud-native, enterprise data integration service for quickly building and managing data pipelines, this scenario is easily surmountable. Along with tools like Cloud Data Loss Prevention (DLP), Google Cloud Storage, and BigQuery, the extraction, transformation, and load activities are addressed.
Google Cloud Data Fusion enables business users to build a data pipeline without writing a line of code. With all of its built-in connectors, creating a pipeline between an S3 and the company's data lake happens with only a few clicks.
The biggest barrier to enterprise data analysis and machine learning is data integration. It is not uncommon to see companies struggle to get their data in one place, move it around, transform it, and make sense of it. Cloud Data Fusion is a fully-managed, cloud-native data integration service that helps users efficiently build and manage data pipelines. Data Fusion shifts an organization's focus away from code and integration to insights and action with its graphical interface and a broad open-sourced library of preconfigured connectors and transformations.
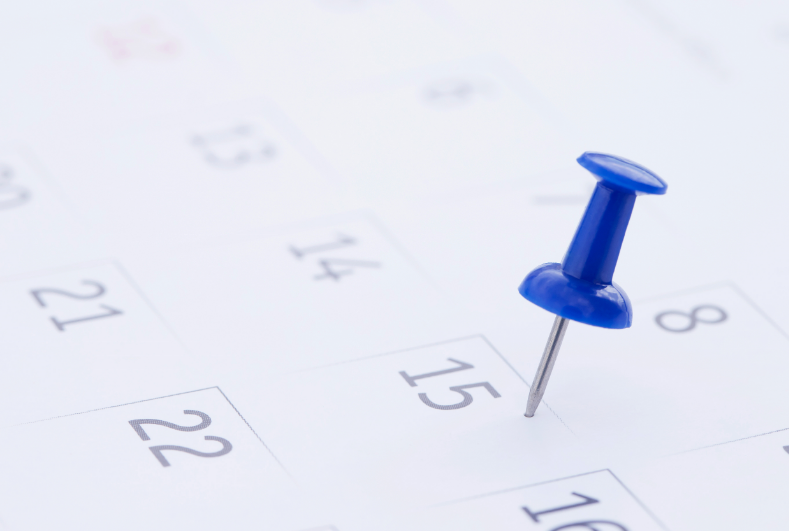
To see how you can easily consolidate your data streams into actionable insights, Consult an expert today. WALT Lab's team of cloud experts can show you how to consolidate your data and create better insights easily.